System integration, storage, and grid in WITCH
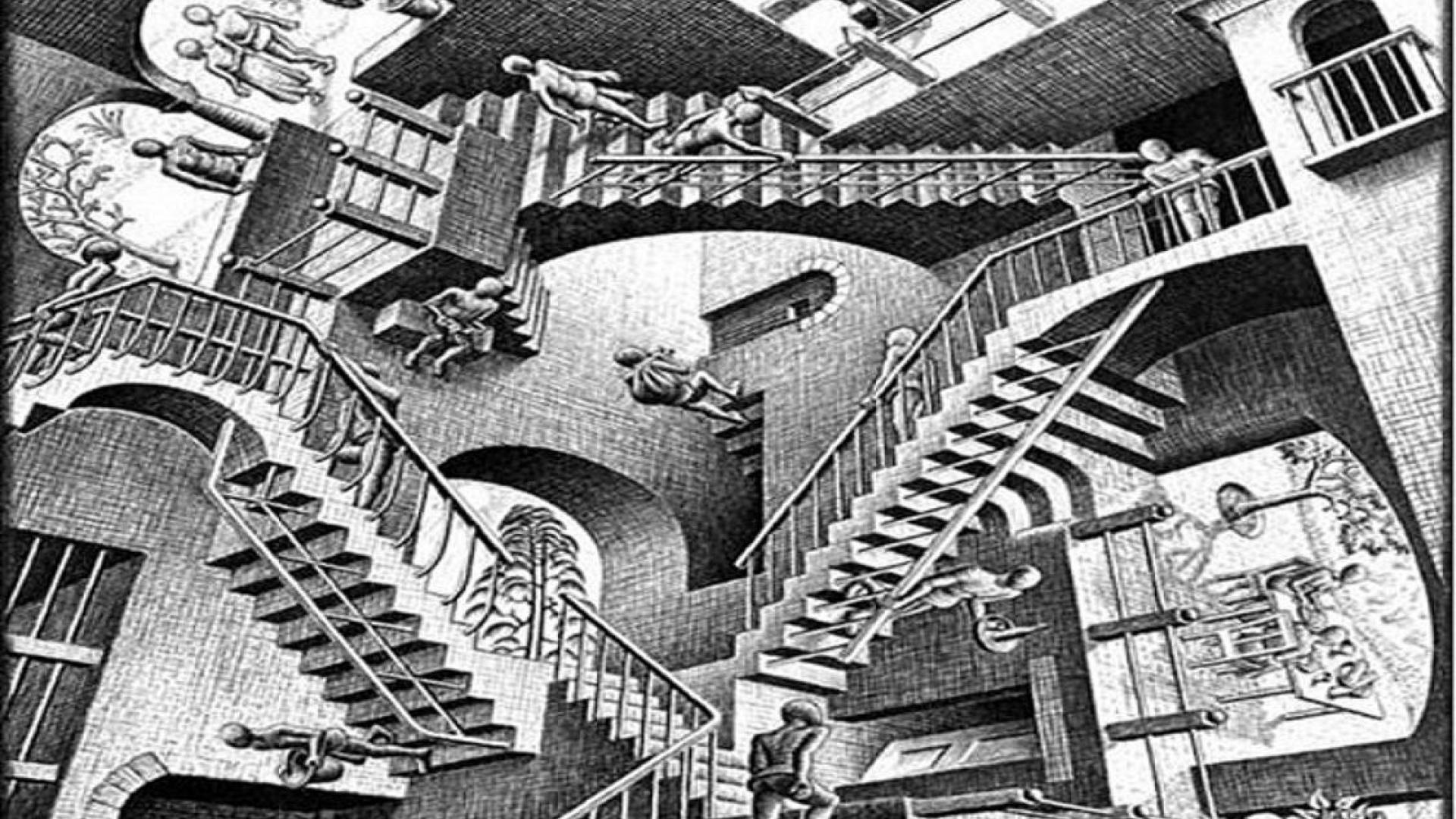
Last January I finally started the outgoing phase of my Marie Skłodowska-Curie Global Fellowship at the University of California, Berkeley. Finally, because I really chased this fellowship for years, as illustrated in this short cartoon.
Why Berkeley? At UC Berkeley, you can find the Renewable & Appropriate Energy Laboratory (RAEL) – directed by Prof. Daniel Kammen – which is leader in the field of energy modeling and thus an ideal place to develop the first part of my fellowship, dedicated to the “Power sector modeling improvements” of the WITCH model. The idea is to carry out these modeling improvements referring to the detailed bottom-up optimization model of the power sector named SWITCH, developed here at UC Berkeley.
These scheduled modeling developments of WITCH regard four aspects, listed here also in order of priority:
- system integration
- electricity storage
- electrical grid
- electricity trade
Concerning system integration, i.e. the issues related to the non-negligible penetration of Variable Renewable Energies in the grid, the objective is firstly to align WITCH to the state-of-the-art modeling as resulted from the EU-FP7 ADVANCE project. After the preliminary literature review that I conducted in the very first weeks of my fellowship, I decided to follow the path of the MESSAGE model. This model implements a modeling mechanism based on two main constraints which are already considered in the current version of WITCH. The first one, named flexibility constraint, requires that the annual average energy production should be flexible enough to be borne by the grid. In other words, the generation fleet must feature enough flexible technologies, such as storage, hydro and gas, to compensate the inflexible ones, i.e. wind and solar. The second one, called capacity constraint, guarantees that the installed firm capacity is sufficient to meet the instantaneous peak capacity demand.
In its new version, the MESSAGE model indirectly uses the Residual Load Duration Curves developed during the ADVANCE research project to refine the formulation of these two constraints. Residual Load Duration Curves are the residual load that results from the subtraction of Variable Renewable Energies generation from Load Duration Curves and should therefore be supplied by dispatchable technologies.
The modeling framework used within MESSAGE was deemed to be a very effective way to implement in WITCH as well the information richness included in the Residual Load Duration Curves, assuring at the same time modeling simplicity and manageability. In fact electricity can still be treated as a homogeneous good over the year, while Residual Load Duration Curves typically consider different load segments (such as peak load, intermediate load, base load, with a possible additional differentiation).
Thus, the WITCH model now considers the updated versions of the capacity constraint (firm capacity requirement and capacity value of wind and solar PV) and the flexibility constraint (operating reserve coefficients for Variable Renewable Energies and load and representation of flexible thermoelectric plant operation), as well as the curtailment of Variable Renewable Energies overproduction.
For what concerns storage and grid, these are already modeled in WITCH, but in a simplified way.
Storage is essentially modeled as an undefined technology, that is helpful to deal with the flexibility and capacity constraints, but that does not directly appear in the electricity system. The grid system is described through a capital equation, which is not linked to the flexibility and capacity constraints.
Currently, I have been working on the planned improvement of the storage modeling in WITCH. The plan is firstly to integrate the storage technology in the electricity production system, describing the charge and discharge phases from and to the grid, also taking into consideration curtailment from the plants fed by Variable Renewable Energies. We will also introduce technology differentiation for storage, allowing for competition between i.e. Pumped Hydroelectric Storage, Compressed Air Energy Storage, and batteries. A similar plan regards the modeling of the grid system, which will be enriched in modeling detail and will be linked to the other parts of the modeling framework in the last part of 2017.
During this year spent at UC Berkeley, I also continued to work as coordinator in a multi-model exercise focused on the modeling of learning in solar PV power plants in Integrated Assessment Models. In addition to WITCH, the participant models are IMAGE, POLES, and REMIND.
In IAMs, the cost evolution of renewable technologies is normally modeled through a learning curve, which yields the capital cost reductions as a function of dedicated R&D investments and/or actual capacity deployment. The objective of the exercise is precisely to explore different cost pathways associated to different learning rates, analyzing how they influence the penetration of solar PV in the electricity mix, and ultimately the overall electricity mix itself. During the past year, the participating teams progressively submitted their results, after which I carried out an integrated analysis of the outcomes, that I have then shared with my partners. The analysis highlighted the need for some corrections in the model scenarios run by the modeling teams and for some additions in the scenario protocol: we have now been working on the changes and improvements for the second and final submission.
In the final months of my fellowship abroad, I will continue to work on the improvements to the storage and grid modeling in WITCH and PV learning in IAMs, while optimizing the possible interactions between WITCH and SWITCH. If you are interested in these research activities, just follow me on my website!
*All the research work illustrated in this article is performed under the project named “MERCURY – Modeling the European power sector evolution: low-carbon generation technologies (renewables, CCS, nuclear), the electric infrastructure and their role in the EU leadership in climate policy” (EC GA No. 706330 – CUP No. E42F16000280006).